Principal Component Analysis#
import numpy as np
import scipy.linalg as la
from sklearn import datasets
import matplotlib.pyplot as plt
Let \(\mathbf{x}_1,\dots,\mathbf{x}_n \in \mathbb{R}^p\) such that the average of the points is located at the origin
Principal component analysis seeks an orthonormal basis \(\mathbf{w}_1 , \dots , \mathbf{w}_p\) such that \(\mathbf{w}_1\) captures the most information (ie. the most variance of the data), and \(\mathbf{w}_2\) captures the most information orthogonal to \(\mathbf{w}_1\) and so on.
Let \(X\) be the \(n \times p\) data matrix with rows \(\mathbf{x}_1,\dots,\mathbf{x}_n\). The weight vectors \(\mathbf{w}_1 , \dots , \mathbf{w}_p\) are the (right) singular vectors of \(X\). That is, the columns of the matrix \(Q\) in the singular value decomposition \(X = P \Sigma Q^T\).
2D Fake Data#
N = 200
x = 4*np.random.randn(N)
y = 0.75*np.random.randn(N)
X = np.column_stack((x,y))
X = X - X.mean(axis=0)
theta = np.pi/6
R = np.array([[np.cos(theta),np.sin(theta)],[-np.sin(theta),np.cos(theta)]])
X = X@R
plt.scatter(X[:,0],X[:,1],alpha=0.5,lw=0)
plt.grid(True); plt.axis('equal'); plt.show();
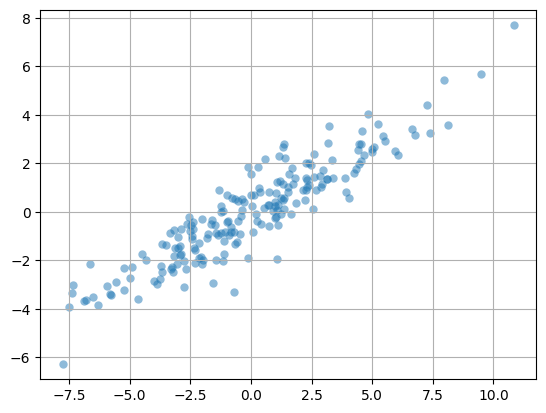
Compute the SVD of the data matrix:
P,S,QT = la.svd(X)
The weight vectors are the rows of \(Q^T\):
QT
array([[ 0.86714106, 0.49806262],
[ 0.49806262, -0.86714106]])
plt.scatter(X[:,0],X[:,1],alpha=0.5,lw=0)
plt.quiver(QT[0,0],QT[0,1],angles='xy',scale_units='xy',scale=0.2)
plt.quiver(QT[1,0],QT[1,1],angles='xy',scale_units='xy',scale=0.2)
plt.grid(True); plt.axis('equal'); plt.show();
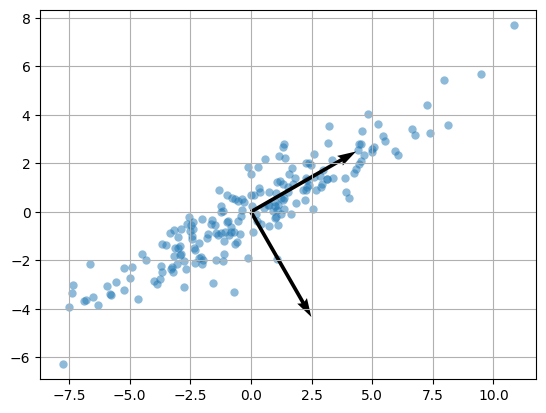
Digits dataset#
sklearn is the Python package for machine learning. There are efficient implementations of algorithms such as PCA included in sklearn however let us use the function scipy.linalg.svd
.
The digits dataset includes 1797 images of handwritten digits. Let’s import the data.
digits = datasets.load_digits()
D = digits['data']
D.shape
(1797, 64)
The matrix \(D\) has 1797 rows and 64 columns. Each row is a 8 by 8 pixel image which has been flattened into a row vector of length 64. Select a row, reshape into an 8 by 8 matrix and plot the image.
plt.imshow(D[1056,:].reshape(8,8),cmap='binary',interpolation='gaussian')
plt.axis(False); plt.show();
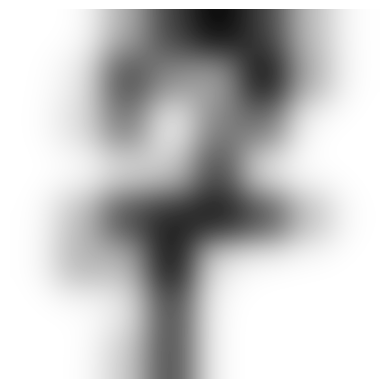
Normlize the matrix by computing the mean of each column and subtracting the result from every row.
X = D - D.mean(axis=0)
Compute the SVD and plot the first weight vector.
P,S,QT = la.svd(X)
plt.imshow(QT[0,:].reshape(8,8),cmap='binary',interpolation='gaussian')
plt.axis(False); plt.show()
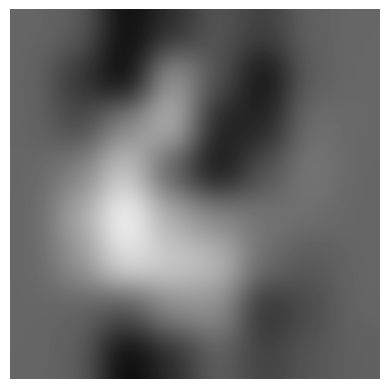
plt.imshow(QT[1,:].reshape(8,8),cmap='binary',interpolation='gaussian')
plt.axis(False); plt.show()
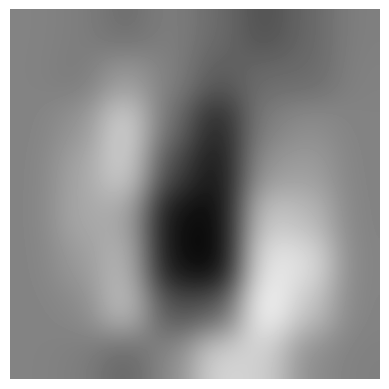
Project the normalized data matrix onto the first two weight vectors.
X2d = X @ QT[0:2,:].T
plt.figure(figsize=(12,8))
plt.scatter(X2d[:,0],X2d[:,1],c=digits['target'],cmap=plt.cm.get_cmap('jet', 10))
plt.colorbar(ticks=range(0,10)); plt.clim([-0.5,9.5]);
plt.show()
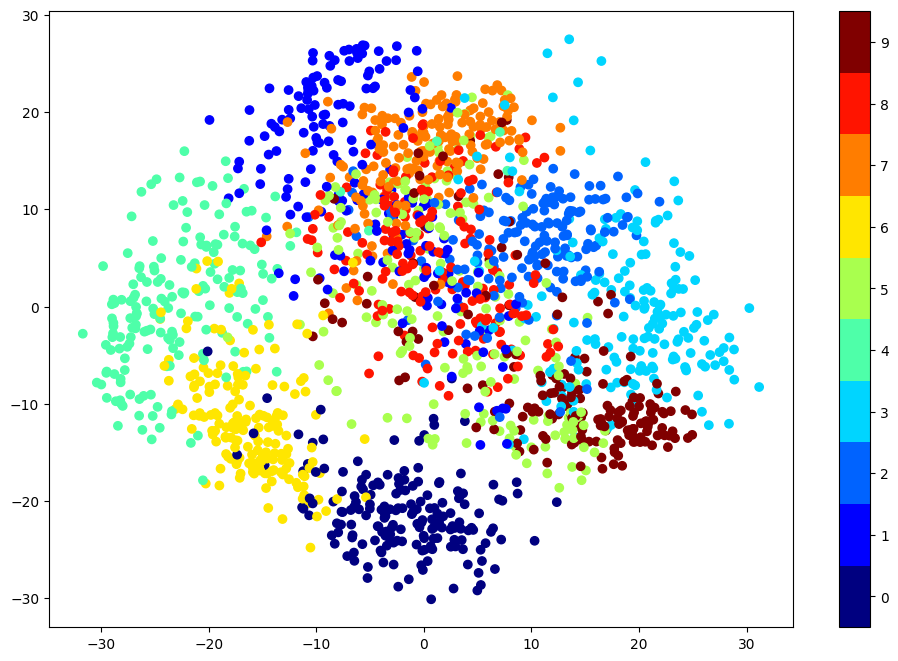