Subspaces#
Subspaces of \(\mathbb{R}^n\) include lines, planes and hyperplanes through the origin. A basis of a subspace is a linearly independent set of spanning vectors. The Rank-Nullity Theorem describes the dimensions of the nullspace and range of a matrix.
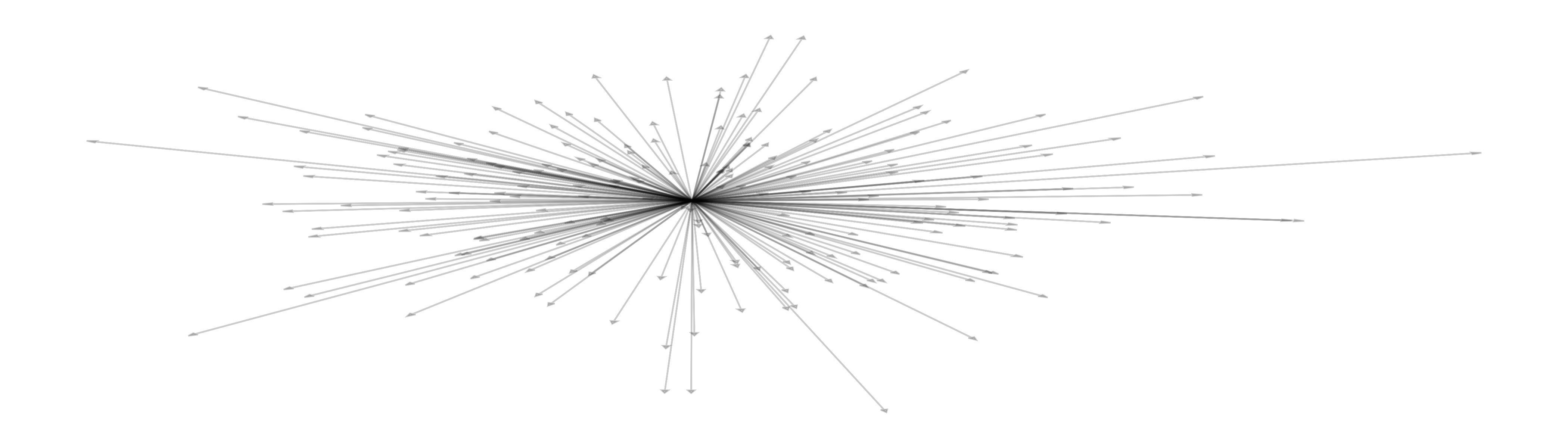
Subspaces#
A subset \(U \subseteq \mathbb{R}^n\) is a subspace if:
\(U\) contains the zero vector \(\boldsymbol{0}\)
\(\boldsymbol{u}_1 + \boldsymbol{u}_2 \in U\) for all \(\boldsymbol{u}_1,\boldsymbol{u}_2 \in U\)
\(c \boldsymbol{u} \in U\) for all \(c \in \mathbb{R},\boldsymbol{u} \in U\)
Condition 2 is called closed under addition. Condition 3 is called closed under scalar multiplication. Condition 3 with \(c=0\) implies Condition 1.
The zero subspace \(\{ \boldsymbol{0} \}\) and the entire space \(\mathbb{R}^n\) are both subspaces of \(\mathbb{R}^n\).
Subspaces of \(\mathbb{R}^2\) include any line through the origin.
Subspaces of \(\mathbb{R}^3\) include any line or plane through the origin.
In general, subspaces of \(\mathbb{R}^n\) are hyperplanes of any dimension through the origin.
Consider the set
Then \(U\) contains the zero vector
and \(U\) is closed under addition since
because \(y_1 + y_2 \geq 0\) since \(y_1 \geq 0\) and \(y_2 \geq 0\). However \(U\) is not closed under scalar multiplication because
Therefore \(U\) is not a subspace of \(\mathbb{R}^2\).
Linear Independence and Span#
A linear combination of vectors \(\boldsymbol{u}_1,\dots,\boldsymbol{u}_m \in \mathbb{R}^n\) is a vector
where \(c_1,\dots,c_m \in \mathbb{R}\). The span of vectors \(\boldsymbol{u}_1,\dots,\boldsymbol{u}_m \in \mathbb{R}^n\) is the set of all linear combinations
Let \(\boldsymbol{u}_1 , \dots , \boldsymbol{u}_m \in \mathbb{R}^n\). Then \(\mathrm{span} \{ \boldsymbol{u}_1 , \dots , \boldsymbol{u}_m \}\) is a subspace of \(\mathbb{R}^n\).
The span of a single nonzero vector \(\boldsymbol{u}\) is a line with direction \(\boldsymbol{u}\). The span of two nonzero vectors \(\boldsymbol{u}\) and \(\boldsymbol{v}\) is a plane as long as \(\boldsymbol{u}\) and \(\boldsymbol{v}\) are not colinear.
A set of vectors \(\{ \boldsymbol{u}_1,\dots,\boldsymbol{u}_m \} \subset \mathbb{R}^n\) forms a linearly independent set if the vectors satisfy the property:
In other words, \(\{ \boldsymbol{u}_1,\dots,\boldsymbol{u}_m \}\) is a linearly independent set if no vector in the set can be expressed as a linear combination of the others.
How do we know if a set of vectors \(\{ \boldsymbol{u}_1,\dots,\boldsymbol{u}_m \}\) is linearly independent? Create a matrix where the columns are the given vectors
Then \(\{ \boldsymbol{u}_1,\dots,\boldsymbol{u}_m \}\) is a linearly independent set if and only if the linear system \(A \boldsymbol{x} = \boldsymbol{0}\) has only the trivial solution \(\boldsymbol{x} = \boldsymbol{0}\).
Basis and Dimension#
Let \(U \subseteq \mathbb{R}^n\) be a subspace. A set of vectors \(\{ \boldsymbol{u}_1 , \dots , \boldsymbol{u}_m \}\) forms a basis of \(U\) if:
\(\{ \boldsymbol{u}_1 , \dots , \boldsymbol{u}_m \}\) is a linearly independent set
\(\mathrm{span} \{ \boldsymbol{u}_1 , \dots , \boldsymbol{u}_m \} = U\)
The dimension of \(U\) is the number \(m\) of vectors in a basis.
Nullspace and Range#
The nullspace of a \(m \times n\) matrix \(A\) is
Let \(A\) be a \(m \times n\) matrix. The nullspace \(N(A)\) is a subspace of \(\mathbb{R}^n\).
Let \(A\) be a \(m \times n\) matrix and let \(A = LU\) be the LU decomposition (if it exists). Then \(N(A) = N(U)\).
The range of a \(m \times n\) matrix \(A\) is:
The range of \(A\) is also called image or column space of \(A\).
Matrix multiplication can be written as
Therefore the range of \(A\) is the equal to the span of the columns
and that’s why \(R(A)\) is sometimes called the column space.
Let \(A\) be a \(m \times n\) matrix. The range \(R(A)\) is a subspace of \(\mathbb{R}^m\).
Let \(A\) be an \(m \times n\) matrix. Then
Proof. The rank of \(A\) is the number of nonzero rows in the row echelon form of \(A\). The dimension of \(R(A)\) is the number of linearly independent columns in \(A\) which is also equal to the number of nonzero rows in \(A\).
Let \(A = LU\) be the LU decomposition of \(A\) (if it exists) and let \(r = \mathrm{rank}(A)\). Then
where \(\boldsymbol{\ell}_1 , \dots , \boldsymbol{\ell}_r\) are the first \(r\) columns of \(L\). In particular, \(\boldsymbol{\ell}_1 , \dots , \boldsymbol{\ell}_r\) is a basis of \(R(A)\).
Proof. If \(\mathrm{rank}(A) = r\) then only the first \(r\) entries of the vector \(U \boldsymbol{x}\) are nonzero
Therefore
Rank-Nullity Theorem#
Let \(A\) be an \(m \times n\) matrix. Then
Proof. The dimension of \(N(A)\) is equal to the number of columns of the row echelon form of \(A\) without a leading nonzero entry, and \(\mathrm{rank}(A) = \dim(R(A))\) is equal to the number of columns of the row echelon form of \(A\) with a leading nonzero entry, and there are \(n\) total columns.
Exercises#
Determine whether or not the set
is a subspace of \(\mathbb{R}^3\).
Solution
\(U\) is not a subspace because it is not closed under vector addition.
Determine whether \(\mathrm{span} \{ \boldsymbol{u}_1 , \boldsymbol{u}_2 \} = \mathrm{span} \{ \boldsymbol{u}_3 , \boldsymbol{u}_4 \}\) where
Solution
\(\mathrm{span} \{ \boldsymbol{u}_1 , \boldsymbol{u}_2 \} \ne \mathrm{span} \{ \boldsymbol{u}_3 , \boldsymbol{u}_4 \}\) since \(\boldsymbol{u}_1,\boldsymbol{u}_2,\boldsymbol{u}_4\) are linearly independent.
Let \(U = \mathrm{span} \{ \boldsymbol{u}_1 , \boldsymbol{u}_2 , \boldsymbol{u}_3 , \boldsymbol{u}_4 \} \subseteq \mathbb{R}^4\) where
Find a basis and the dimension of \(U\).
Is \(\{ \boldsymbol{u}_1 , \boldsymbol{u}_3 , \boldsymbol{u}_4 \}\) a basis of \(U\)? Explain.
Solution
\(\dim(U) = 3\) and \(\{ \boldsymbol{u}_1 , \boldsymbol{u}_3 , \boldsymbol{u}_4 \}\) also forms a basis of \(U\).
Let \(A = LU\) be the LU decomposition of \(A\). Determine whether the statement is True or False.
\(N(A) = N(U)\)
\(\dim (N(A)) = \dim (N(U))\)
\(R(A) = R(U)\)
\(\dim (R(A)) = \dim (R(U))\)
Solution
True
True
False
True