Linear Regression#
import numpy as np
import matplotlib.pyplot as plt
import scipy.linalg as la
import pandas as pd
Linear Regression#
A linear regression model is a function of the form
where \(\mathbf{x} = (x_1,\dots,x_p)\) and \(\boldsymbol{\beta} = (\beta_0,\beta_1,\dots,\beta_p)\).
Least Squares#
Least squares regression corresponds to the case where we choose the sum of squared errors cost function
For simple linear regression this corresponds to
where
Note that \(X \boldsymbol{\beta}\) is a vector in the column space of \(X\) therefore the distance \(|| \mathbf{y} - X \boldsymbol{\beta} ||\) is minimized when the line from \(\mathbf{y}\) to \(X\boldsymbol{\beta}\) is orthogonal to the column space. In other words, we want to find \(\boldsymbol{\beta}\) such that \(X^T(\mathbf{y} - X \boldsymbol{\beta}) = 0\) which leads to the normal equations
Residuals#
The difference between the observed value \(y_i\) and the predicted value \(f(\mathbf{x}_i ; \boldsymbol{\beta})\) is called the residual
Example: Real Estate Prices#
The data below was collected from REALTOR.ca for 2-bedroom apartments for sale in the Kitsilano neighborhood of Vancouver. The dependent variable \(y\) is the listing price and the independent variable \(x\) is the apartment size (in square feet).
x = [1557,1452,767,900,1018,802,924,981,879,819,829,1088,1085]
y = [1.398,1.750,0.899,0.848,1.149,0.825,0.999888,1.175,0.885,0.749888,1.098,1.099,1.198]
plt.plot(x,y,'.')
plt.title('Listing Price on REALTOR.ca')
plt.xlabel('Apartment Size (sqft)'), plt.ylabel('Price (Millons $)'), plt.grid(True)
plt.show()
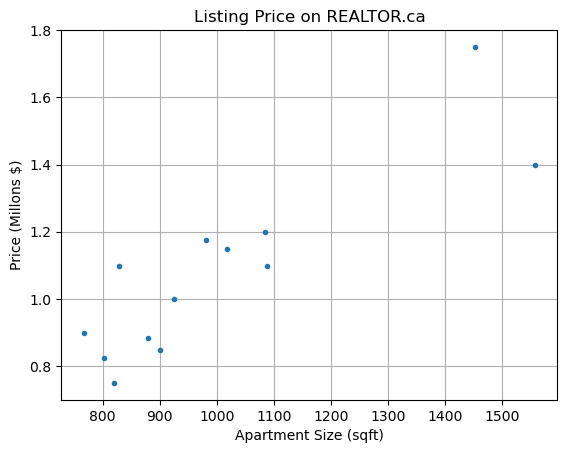
Choose a simple linear regression model for the data \(y = \beta_0 + \beta_1 x + \varepsilon\). Construct the matrix \(X\):
X = np.column_stack([np.ones(len(x)),x])
X
array([[1.000e+00, 1.557e+03],
[1.000e+00, 1.452e+03],
[1.000e+00, 7.670e+02],
[1.000e+00, 9.000e+02],
[1.000e+00, 1.018e+03],
[1.000e+00, 8.020e+02],
[1.000e+00, 9.240e+02],
[1.000e+00, 9.810e+02],
[1.000e+00, 8.790e+02],
[1.000e+00, 8.190e+02],
[1.000e+00, 8.290e+02],
[1.000e+00, 1.088e+03],
[1.000e+00, 1.085e+03]])
Solve the normal equations:
beta = la.solve(X.T@X,X.T@y)
beta
array([0.10620723, 0.00096886])
Plot the linear regression model with the data:
xs = np.linspace(700,1600)
ys = beta[0] + beta[1]*xs
plt.plot(x,y,'.',xs,ys)
plt.title('Listing Price on REALTOR.ca'), plt.grid(True)
plt.xlabel('Apartment Size (sqft)'), plt.ylabel('Price (Millons $)')
plt.show()
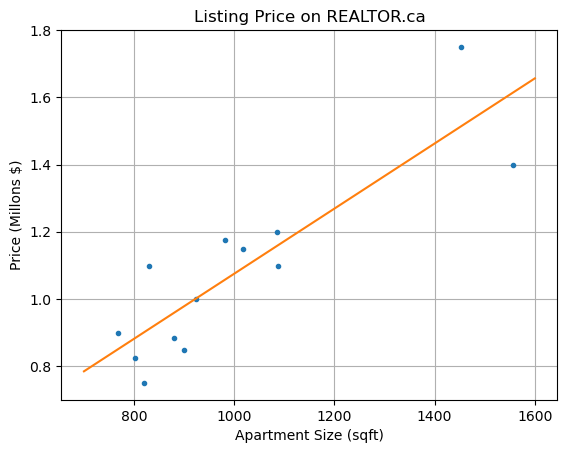
The coefficient \(\beta_1 = 0.00096886\) suggests that the listing price of a 2-bedroom apartment increases by \(968.86\) dollars per square foot.
Example: Temperature#
Vancouver weather data is available at vancouver.weatherstats.ca. Plot the daily average temperature as function of the day of the year.
df = pd.read_csv('temperature.csv')
df.head()
day | month | year | dayofyear | avg_temperature | |
---|---|---|---|---|---|
0 | 13 | 4 | 2023 | 103 | 7.10 |
1 | 12 | 4 | 2023 | 102 | 5.19 |
2 | 11 | 4 | 2023 | 101 | 8.00 |
3 | 10 | 4 | 2023 | 100 | 7.69 |
4 | 9 | 4 | 2023 | 99 | 9.30 |
df.tail()
day | month | year | dayofyear | avg_temperature | |
---|---|---|---|---|---|
9995 | 1 | 12 | 1995 | 335 | 6.40 |
9996 | 30 | 11 | 1995 | 334 | 9.15 |
9997 | 29 | 11 | 1995 | 333 | 11.50 |
9998 | 28 | 11 | 1995 | 332 | 9.75 |
9999 | 27 | 11 | 1995 | 331 | 6.90 |
plt.plot(df['dayofyear'],df['avg_temperature'],'.',alpha=0.1,lw=0)
plt.title('Daily Average Temperature 1995-2023')
plt.xlabel('Day of the Year'), plt.ylabel('Temperature (Celsius)'), plt.grid(True)
plt.show()
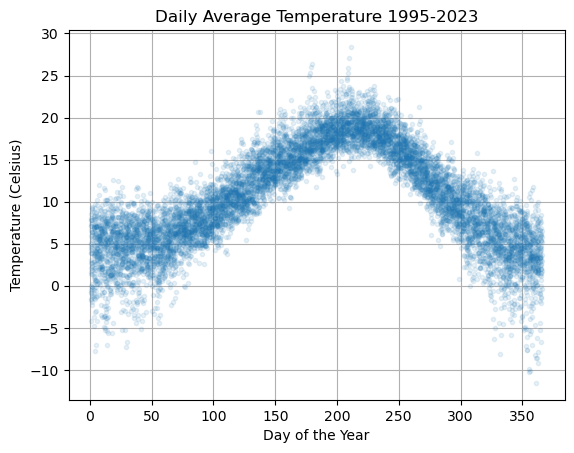
Let \(T\) by the temperature and let \(d\) be the day of the year. Define a regression model of the form
Note that if we rewrite the variables as \(y = T\), \(x_1 = \cos(2 \pi d/365)\) and \(x_2 = \sin(2 \pi d/365)\) then we see that this is indeed a linear regression model
Construct the corresponding data matrix \(X\) and compute the coefficient vector \(\boldsymbol{\beta}\).
N = len(df)
d = df['dayofyear']
T = df['avg_temperature']
X = np.column_stack([np.ones(N),np.cos(2*np.pi*d/365),np.sin(2*np.pi*d/365)])
beta = la.solve(X.T@X,X.T@T)
ds = np.linspace(0,365,500)
Ts = beta[0] + beta[1]*np.cos(2*np.pi*ds/365) + beta[2]*np.sin(2*np.pi*ds/365)
plt.plot(df['dayofyear'],df['avg_temperature'],'.',alpha=0.1,lw=0)
plt.plot(ds,Ts,'r'), plt.grid(True)
plt.title('Daily Average Temperature 1995-2023')
plt.xlabel('Day of the Year'), plt.ylabel('Temperature (Celsius)')
plt.show()
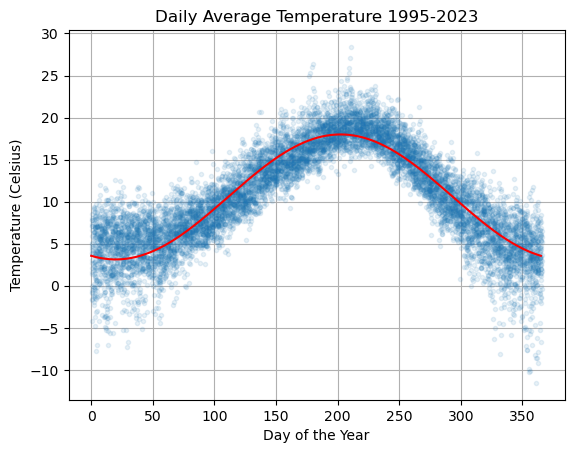
f = lambda d,beta: beta[0] + beta[1]*np.cos(2*np.pi*d/365) + beta[2]*np.sin(2*np.pi*d/365)
residual = T - f(d,beta)
plt.hist(residual,bins=50,density=True)
plt.show()
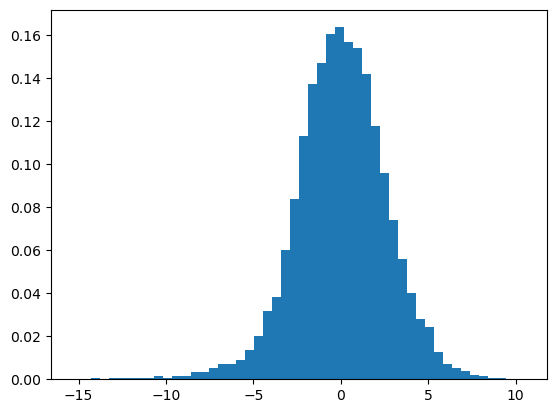
la.norm(residual)/N
0.026025310340052316
Example: Temperature#
We can see in teh previous example that the linear model doesn’t quite fit the data. Let’s modify our linear regression model to include periodic functions with periods of 1 year (365 days) and 6 months (365/2 days):
Construct the corresponding data matrix \(X\) and compute the coefficient vector \(\boldsymbol{\beta}\).
N = len(df)
d = df['dayofyear']
T = df['avg_temperature']
X = np.column_stack([np.ones(N),
np.cos(2*np.pi*d/365),np.sin(2*np.pi*d/365),
np.cos(4*np.pi*d/365),np.sin(4*np.pi*d/365)])
beta = la.solve(X.T@X,X.T@T)
ds = np.linspace(0,365,500)
x1 = np.cos(2*np.pi*ds/365)
x2 = np.sin(2*np.pi*ds/365)
x3 = np.cos(4*np.pi*ds/365)
x4 = np.sin(4*np.pi*ds/365)
Ts = beta[0] + beta[1]*x1 + beta[2]*x2 + beta[3]*x3 + beta[4]*x4
plt.plot(df['dayofyear'],df['avg_temperature'],'.',alpha=0.1,lw=0)
plt.plot(ds,Ts,'r'), plt.grid(True)
plt.title('Daily Average Temperature 1995-2023')
plt.xlabel('Day of the Year'), plt.ylabel('Temperature (Celsius)')
plt.show()
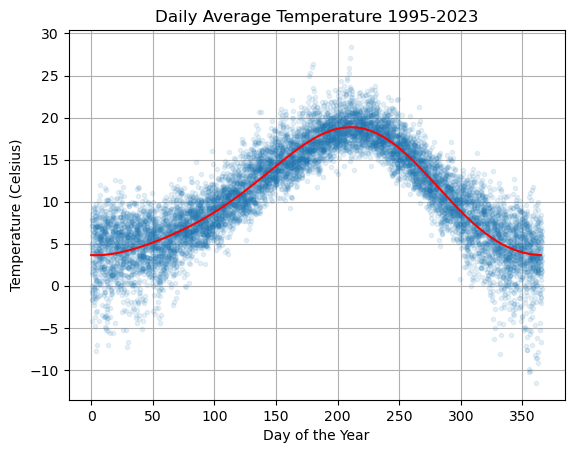
f = lambda d,beta: beta[0] + beta[1]*np.cos(2*np.pi*d/365) + beta[2]*np.sin(2*np.pi*d/365) + beta[3]*np.cos(4*np.pi*d/365) + beta[4]*np.sin(4*np.pi*d/365)
residual = T - f(d,beta)
plt.hist(residual,bins=50,density=True)
plt.show()
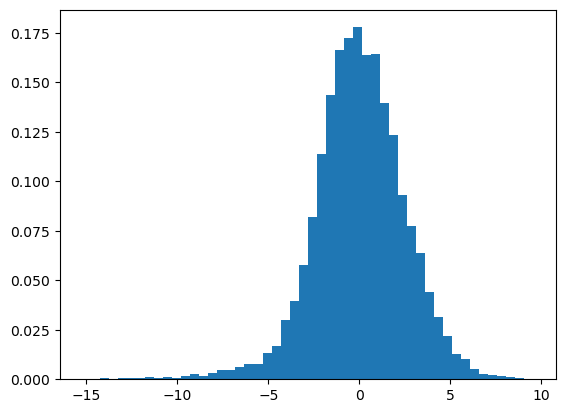
la.norm(residual)/N
0.02490190968939154